Mean classification acc. (%) evaluation of different methods on the MNIST, FMNIST, and CIFAR10 dataset.
MNIST | FMNIST | CIFAR10 | |
---|---|---|---|
FedAvg | 92.62 | 80.58 | 55.65 |
FedProx | 92.57 | 80.50 | 55.10 |
AvgKD | 92.81 | 77.12 | 26.36 |
FedDF | 91.39 | 58.81 | 57.17 |
LG-FedAvg | 92.71 | 80.61 | 54.49 |
GeFL (FedDCGAN) | 95.32 | 83.11 | 58.45 |
GeFL (FedCVAE) | 94.46 | 82.33 | 55.80 |
GeFL (FedDDPMw=0) | 96.44 | 82.43 | 59.36 |
GeFL (FedDDPMw=2) | 95.17 | 81.51 | 58.47 |
Mean classification acc. (%) comparison to data augmentation. GeFL outperforms other baselines and is effective combined with data augmentation.
FedAvg | GeFL (FedDCGAN) | |
---|---|---|
None | 55.65±0.68 | 58.45±0.49 |
MixUp | 60.07±1.13 | 62.67±0.24 |
CutMix | 58.95±0.61 | 61.66±0.41 |
AugMix | 53.96±0.37 | 56.47±0.25 |
AutoAugment | 56.99±0.43 | 59.97±0.38 |
Scalability in client numbers of GeFL and GeFL-F on MNIST, FMNIST, SVHN, and CIFAR dataset. GeFL-F exhibits less performance degradation in a large number of clients compared to GeFL.
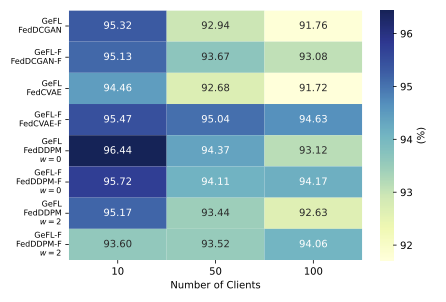
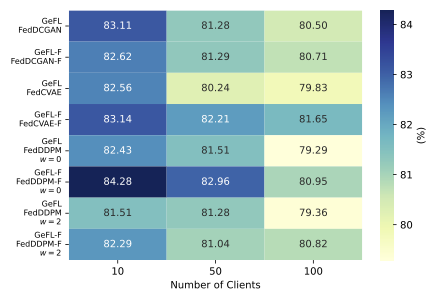
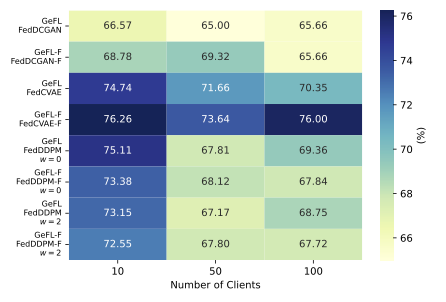
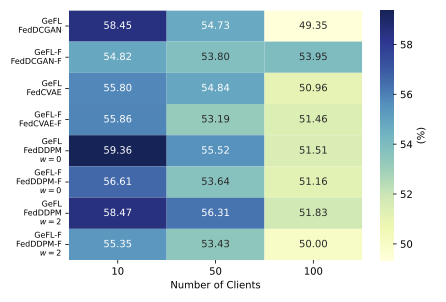
Comparison of privacy, communication and computational costs in GeFL and GeFL-F. Lower values indicate better conditions for each component.